How Pedigree is using AI to transform dog adoption
Nexus Studios’ Technical Director, Vegard Myklebust on using AI tech for good
Nexus Studios teamed up with Colenso BBDO to create Adoptable, an ambitious campaign for Pedigree. By featuring a locally adoptable dog in all of Pedigree’s commercials, we were able to turn every Pedigree advert into an advert for a shelter dog.
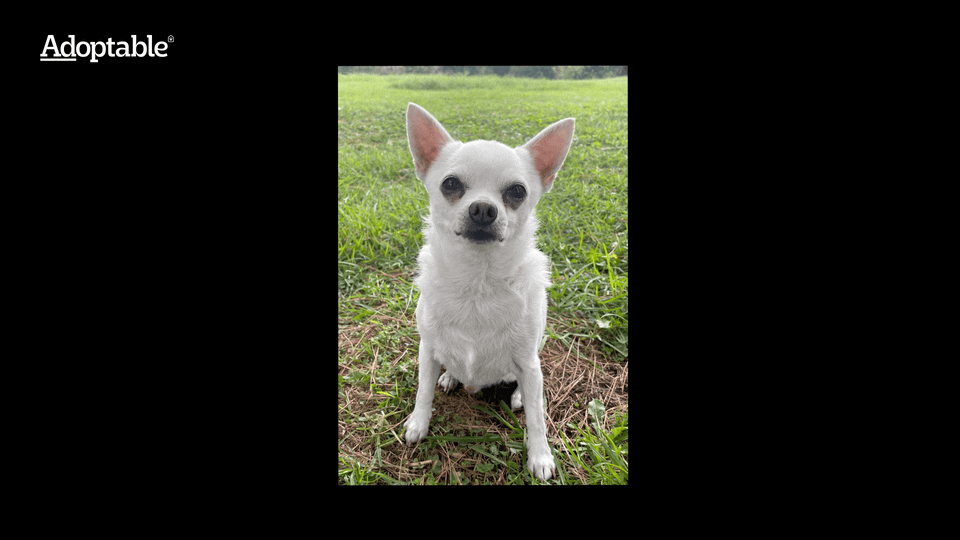
Adoptable’s launch saw a significant uplift in adoption rates. Within the first two weeks, 50% of shelter dogs featured had been adopted, with traffic to shelter site profiles increasing 6 times. Potential pet parents were 12% more likely to adopt when the adopter visits the shelter site via an Adoptable asset, leading Mars to commit to a global rollout.
During judging for the Cannes Outdoor Lions, jury president, Marco Venturelli noted that one of his panel had asserted “We live in a post-purpose world. For me, a post-purpose era is when we don’t jump on things that are fashionable. It is possible for brands to be purposeful in their work at the same time as selling.” In awarding the Cannes Grand Prix – Marco said jurors discussed how the Adoptable campaign was “the right way to take purpose because it is not piggybacking but is at the heart of the business.”
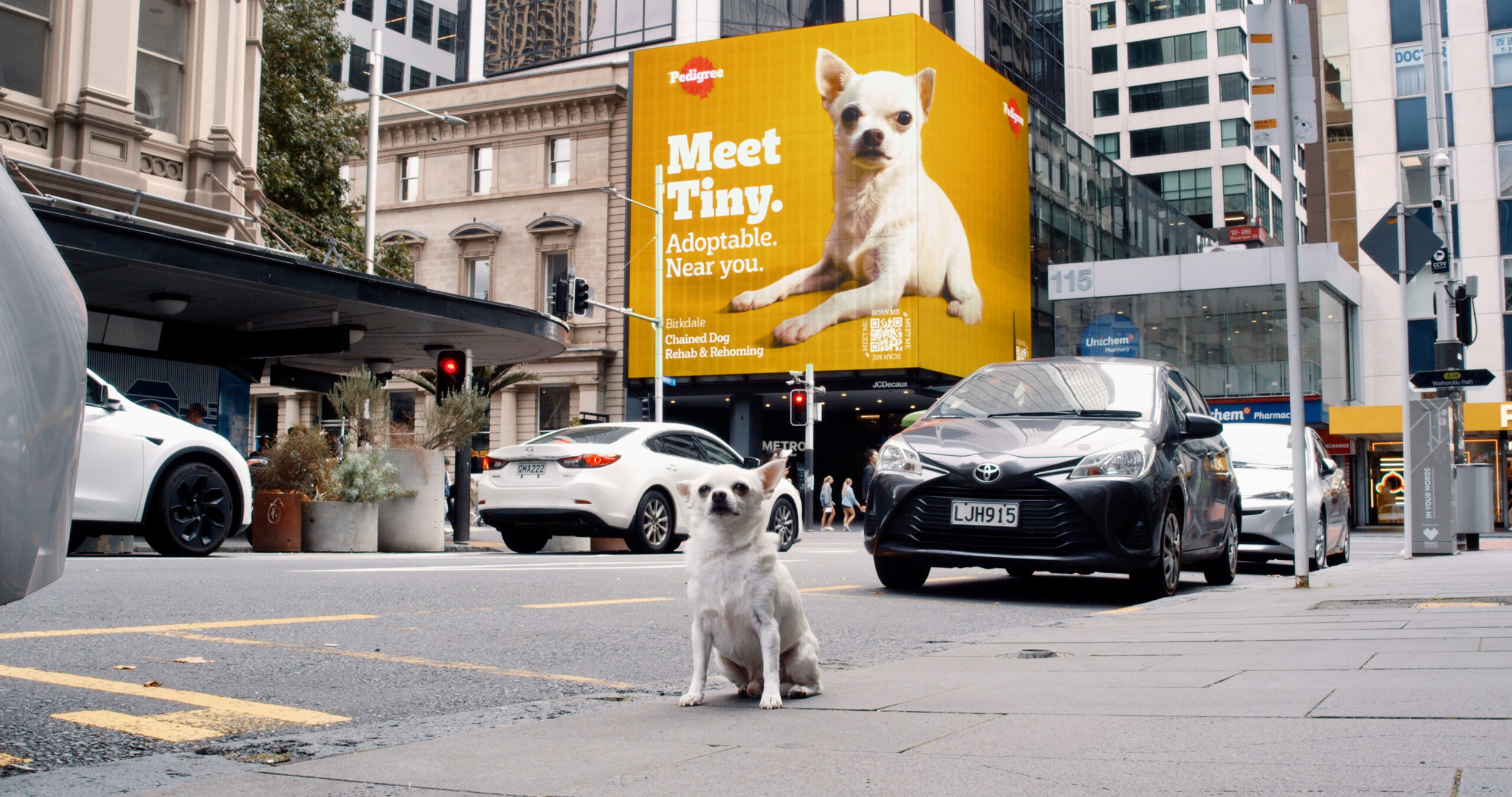
When Colenso BBDO approached us with the Adoptable project for Pedigree, they first thanked us for not dismissing the project as undoable right away. Instead, we worked together with their team to bridge the technological gap between what was achievable right now and what we thought we could do given a bit of time for Research & Development. We decided to approach the problem with a healthy disregard for the impossible, to borrow a slogan from Google’s Larry Page. We knew that 3D was good at making predictable and spatially consistent images, and we knew that generative AI was good at rendering fine detail such as hair, all we had to do was invent a bridge.
“Adoptable is a transformational step for us at Pedigree. We’re committed to helping end dog homelessness around the world.”
– Global Brand VP PEDIGREE at Mars, Fabio Alings
A Smaller Version of the Problem
When a problem is too big to solve, you have to first break down a smaller piece or version of the problem. Dogs have an incredible amount of variation, breed, shape, colour, fur length, markings, are all multipliers for the uniqueness of any particular dog. To make a start, we decided to first focus on a single breed; Chihuahuas. Chihuahuas in themselves are ridiculously varied, and so even with focusing just on one breed, we knew we would bump into some version of just about every problem. We broadly categorised them as Shape Identity, Colour Identity and Fur Identity.
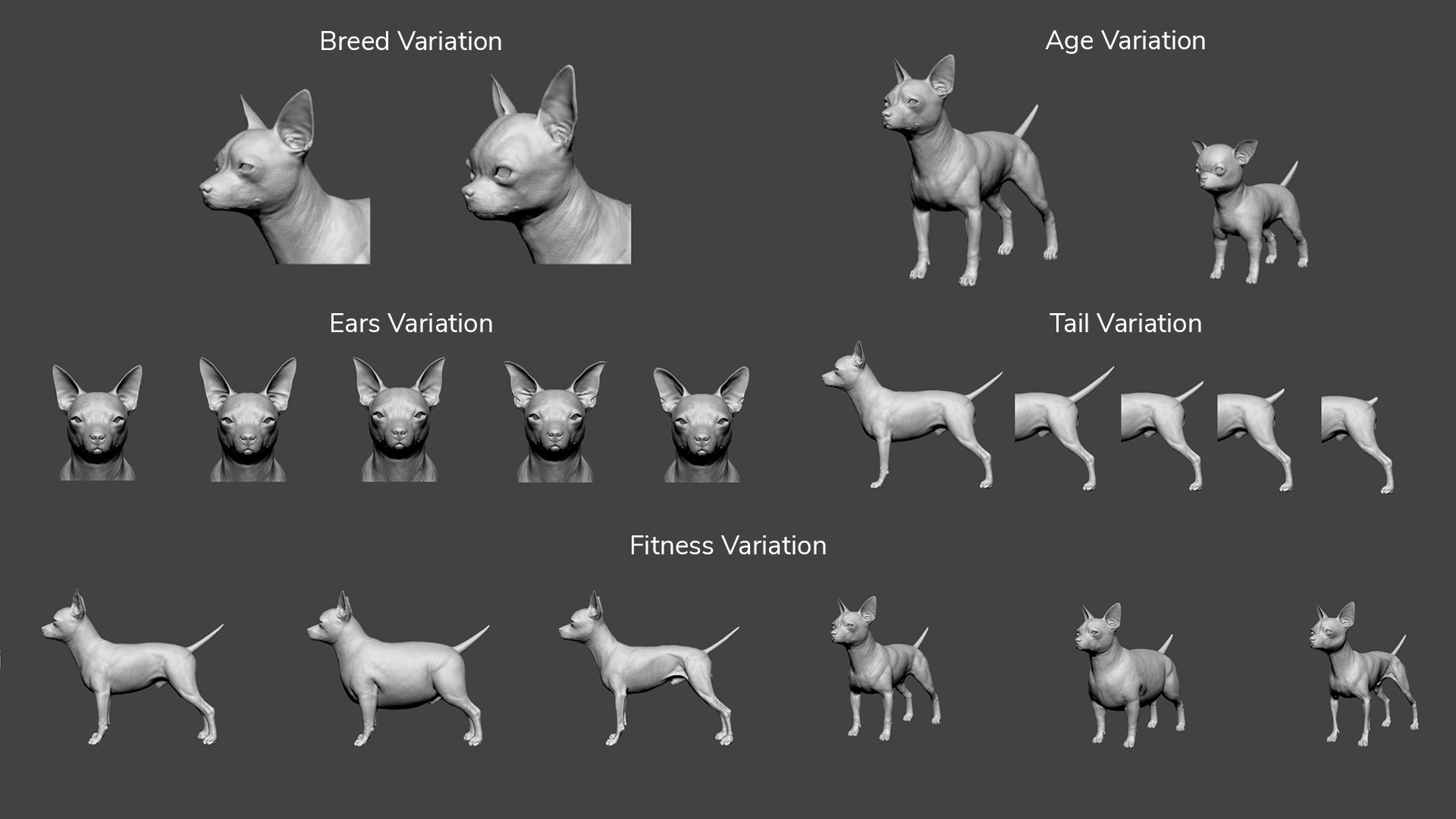
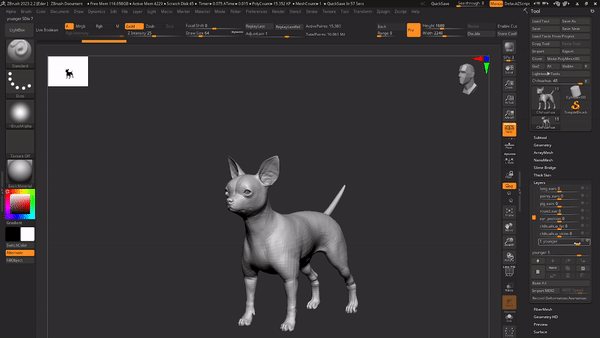
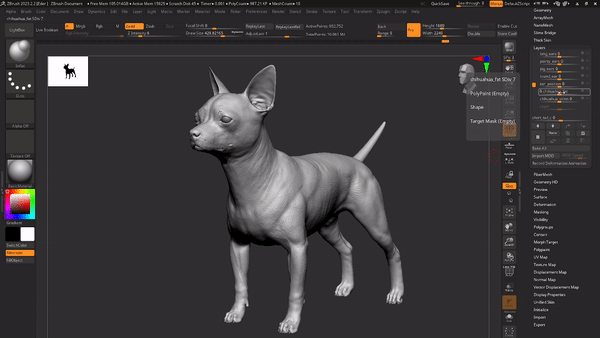
After making the base mesh, we made blend shapes that could be combined to cover a large variety of the Chihuahua’s Shape Identities. Deer head vs apple head, different ages, ears, overall fitness levels and tail lengths etc. Our proprietary digital puppeteering tools are then able to update the rigs based on the resulting shape, so we don’t have to make a new rig or re-pose every dog shape.
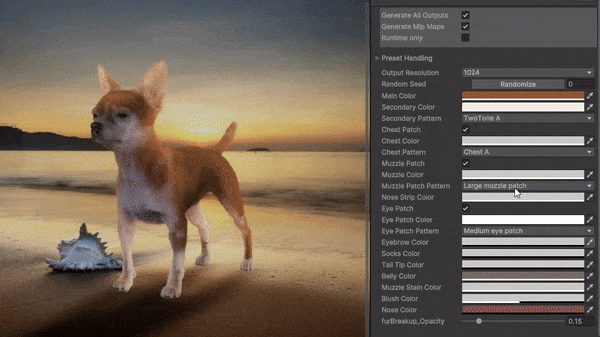
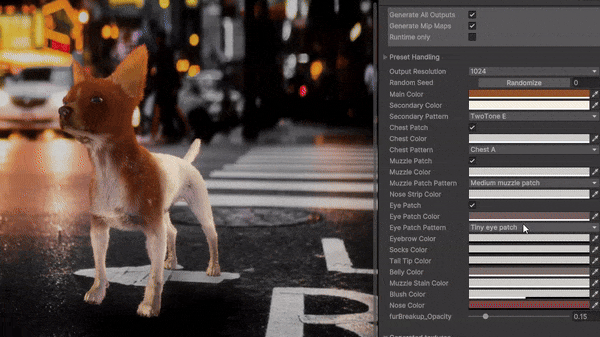
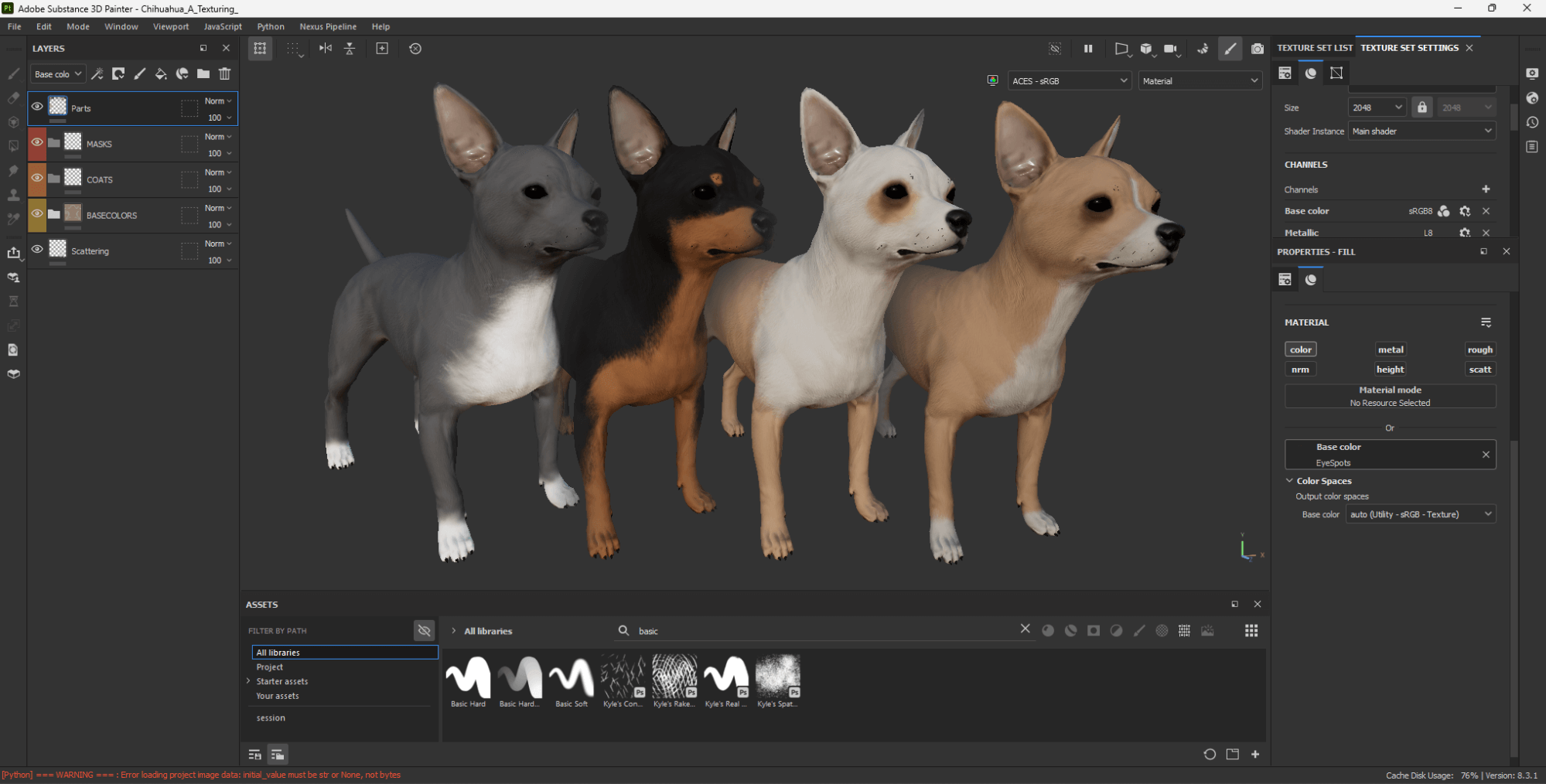
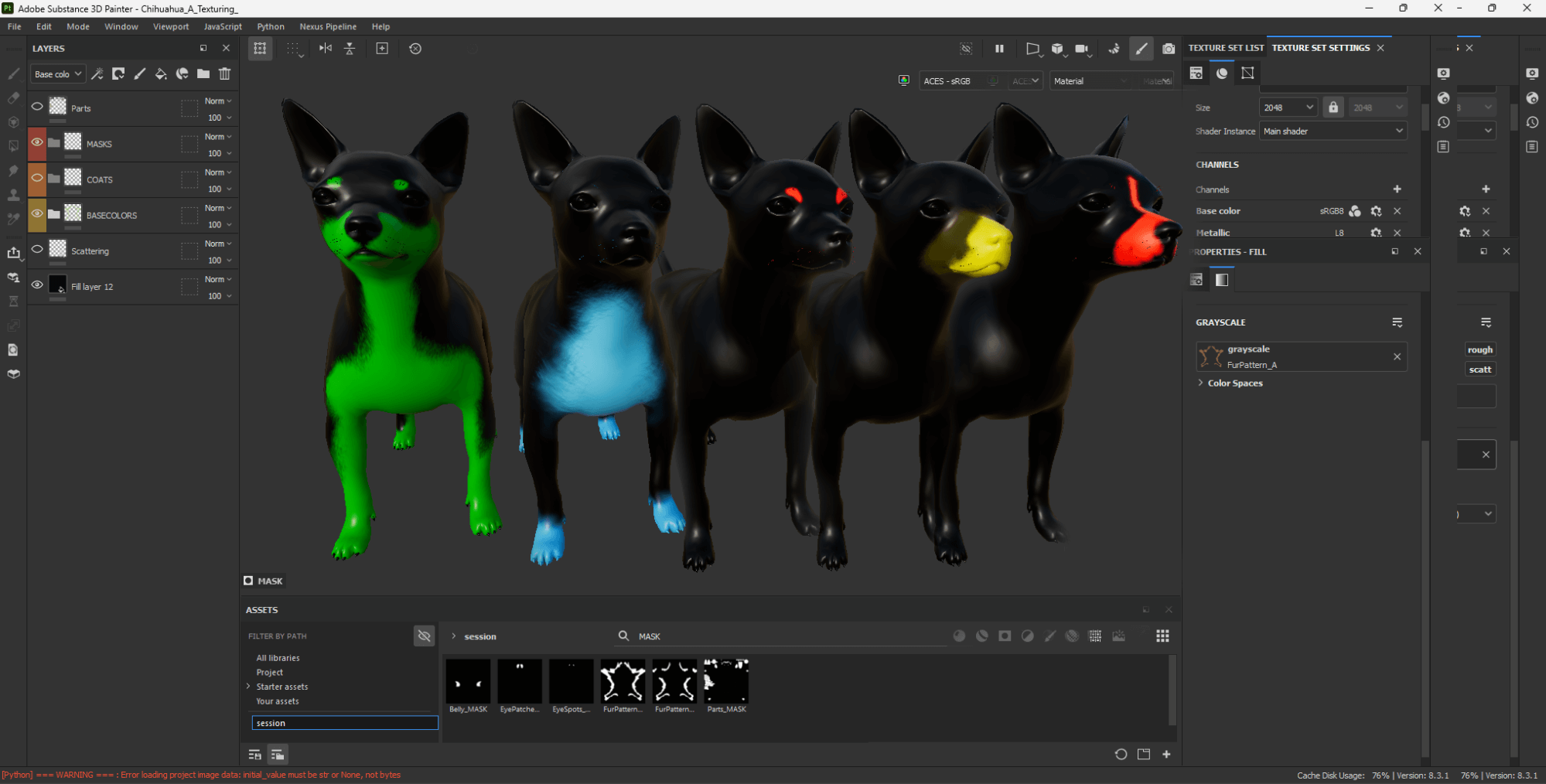
For the Colour Identity, we painted masks to represent different areas that would often have some specific colour variation. Combining the masks and different settings with a procedural material, we were able to unlock a huge amount of variation with fairly simple controls.
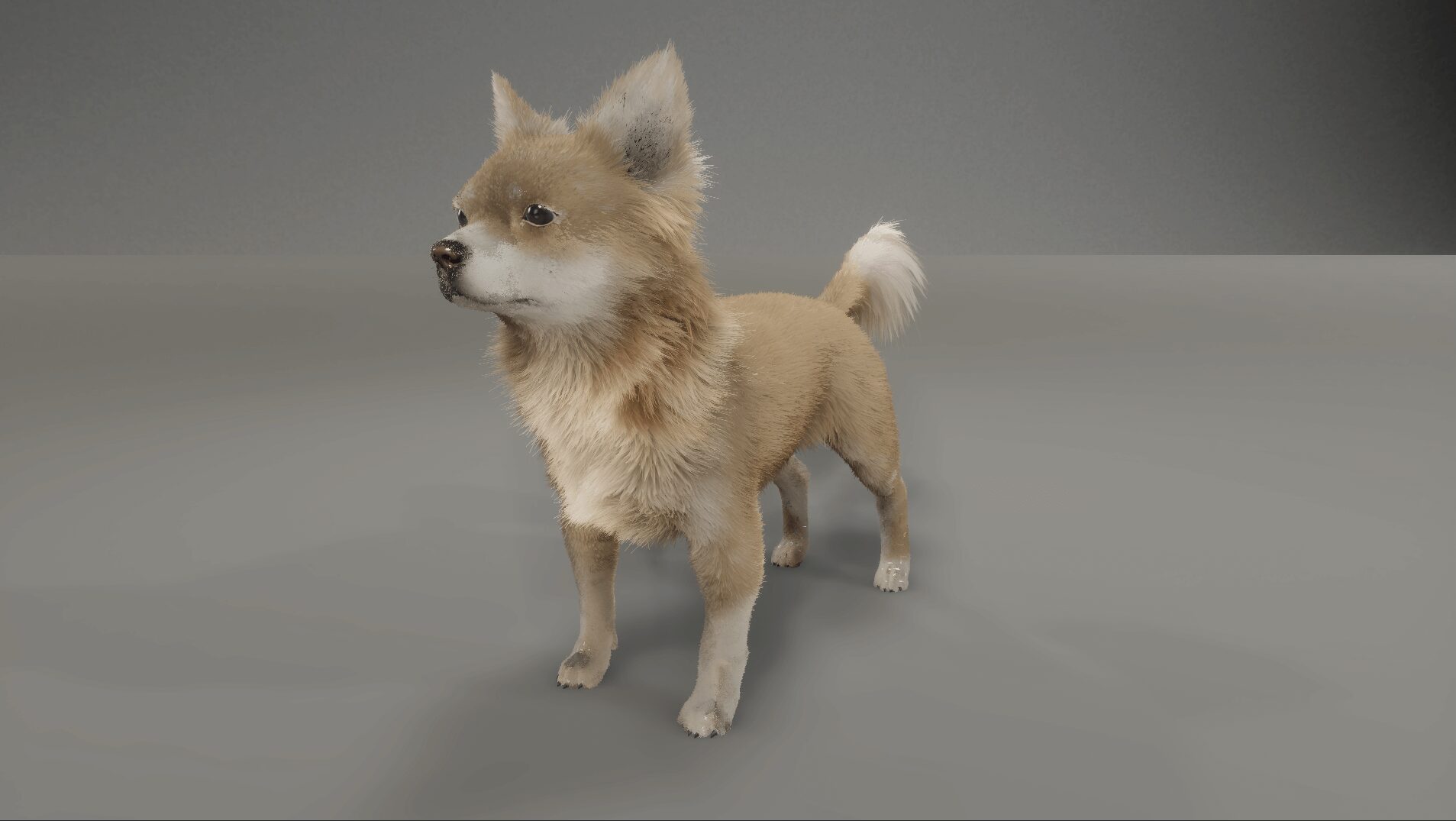
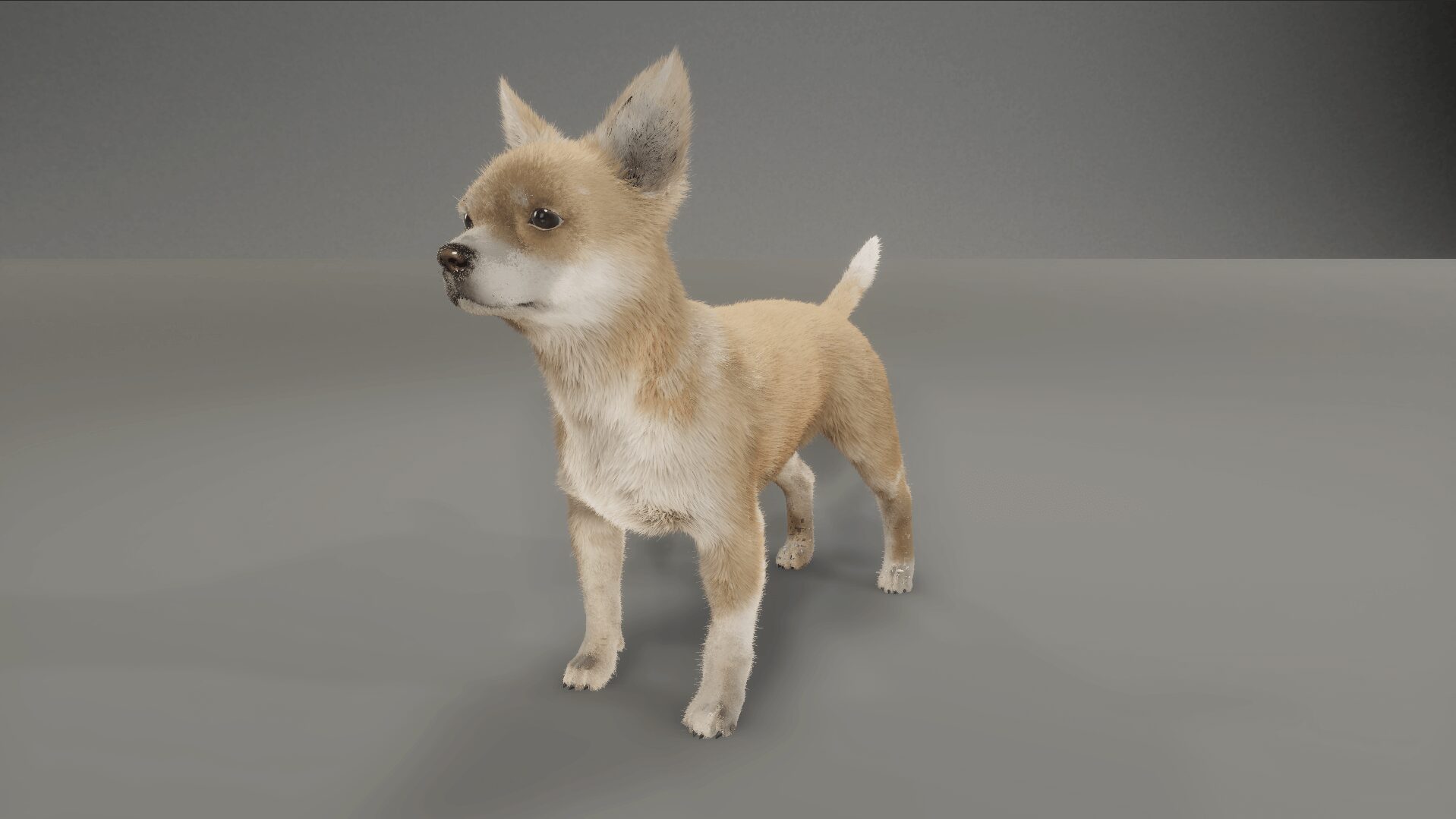
Finally, for the Fur Identity, we settled on making a long groom and a short groom. As with all our other 3D work, we made sure the grooms were blendable, turning the two groom options into a sliding scale rather than just two binary options
With all of these customisable 3D components at the ready, we were able to produce images like you see above in real time that would reflect much of the Shape, Colour and Fur Identity of the dogs.
Housetraining the AI
Meet Pancake, our hero dog for testing.
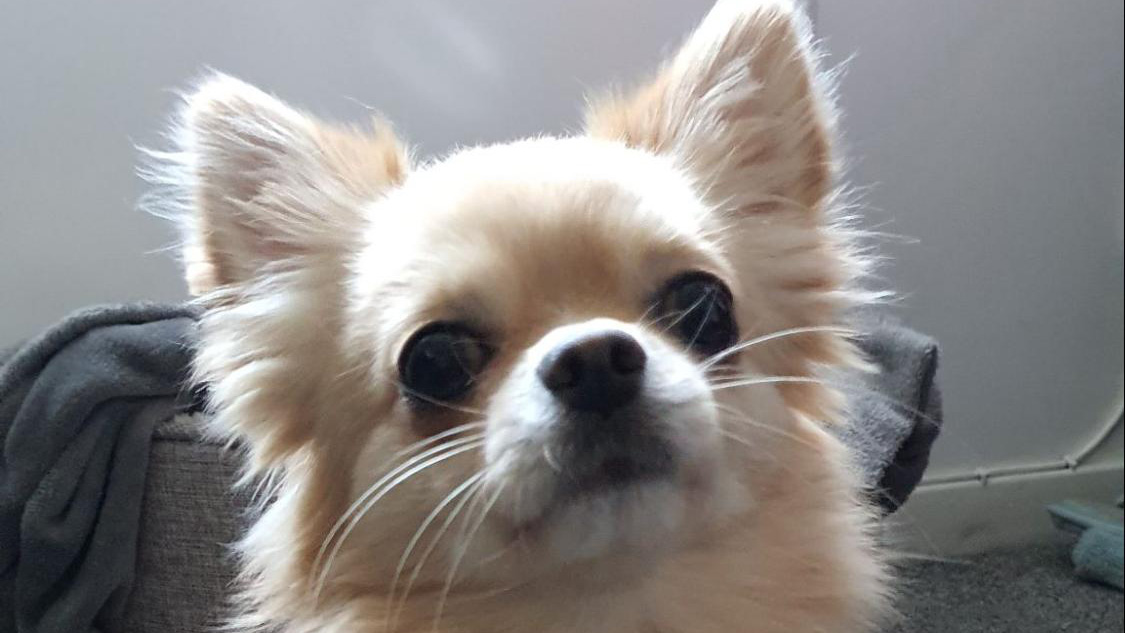
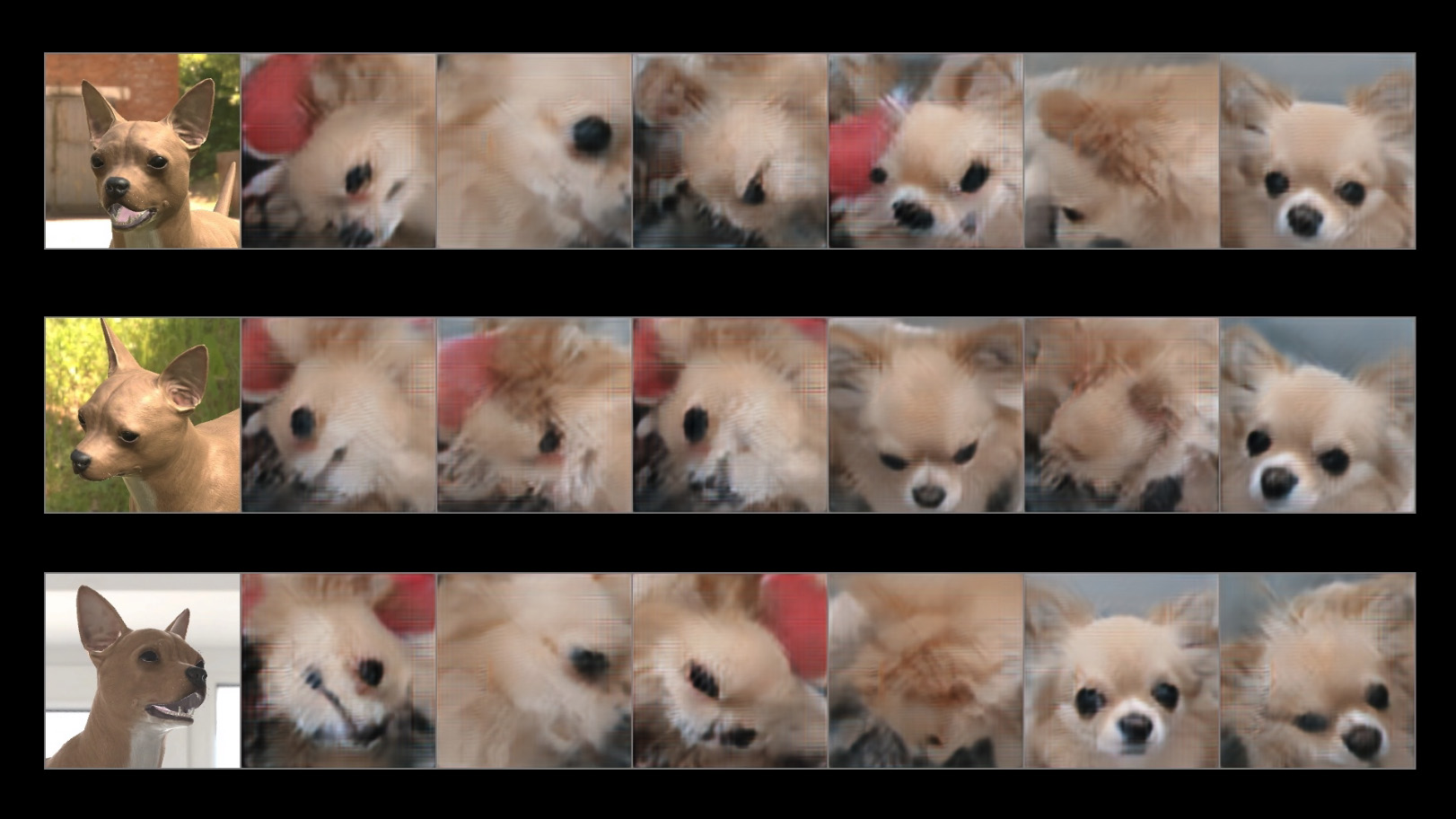
For the AI component, we started by first looking at previous work which was mostly published by NVIDIA using Generative Adversarial Networks. Although we got it to produce dog-like images that had pancake-like features, there were too many limitations to the overall technique to work in production for us, so after a quick bit of investigation, we dropped the GAN techniques in favour of Diffusion models.
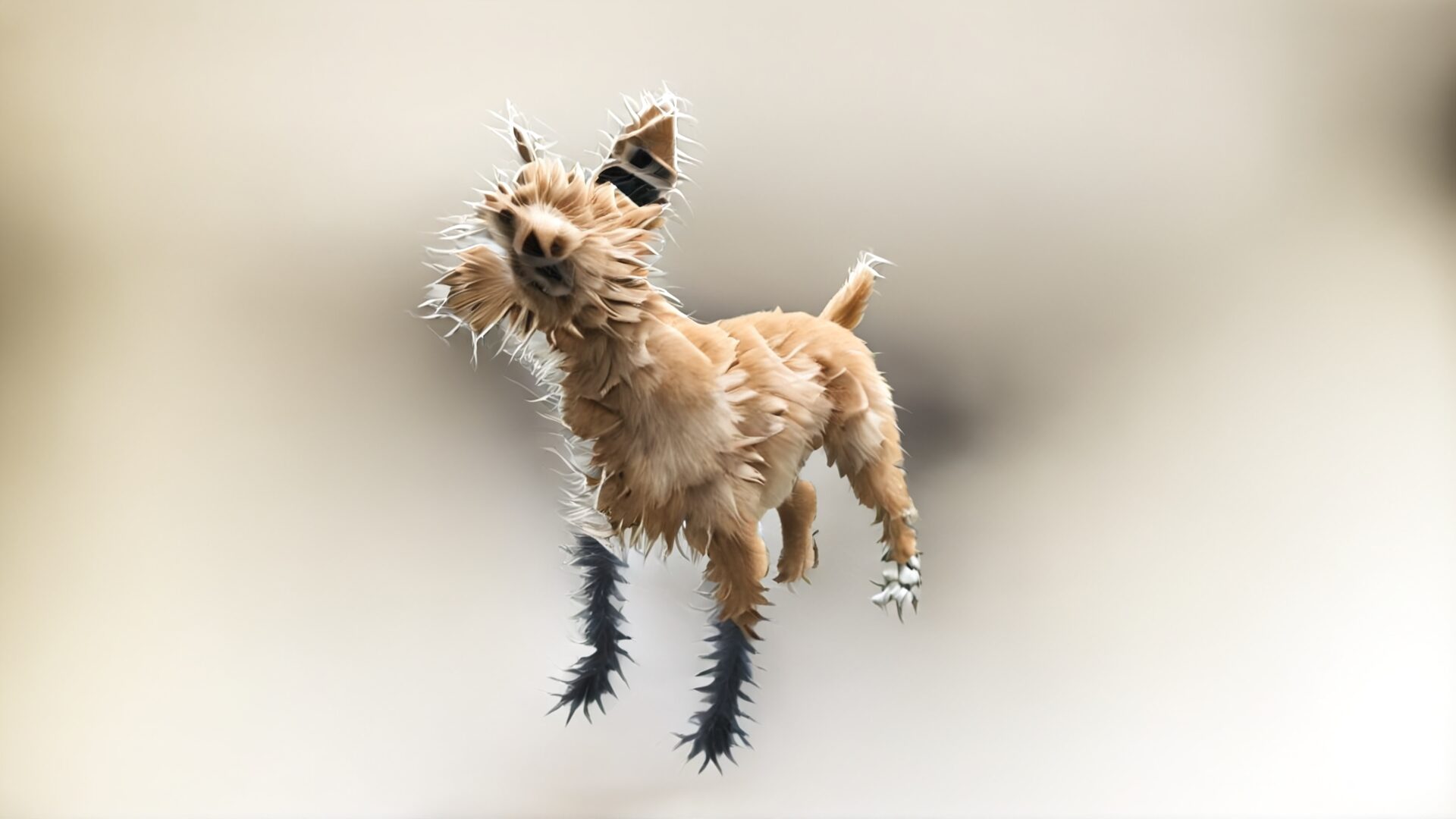
Contrary to how it’s often portrayed, Generative AI doesn’t know too much about how things work, and when you try to instruct it more strongly for what you want, it will often bug out in curious ways. We trained custom small AI models for each dog, experimented with all kinds of Control Nets, and worked on every way of gaining control while improving fidelity.
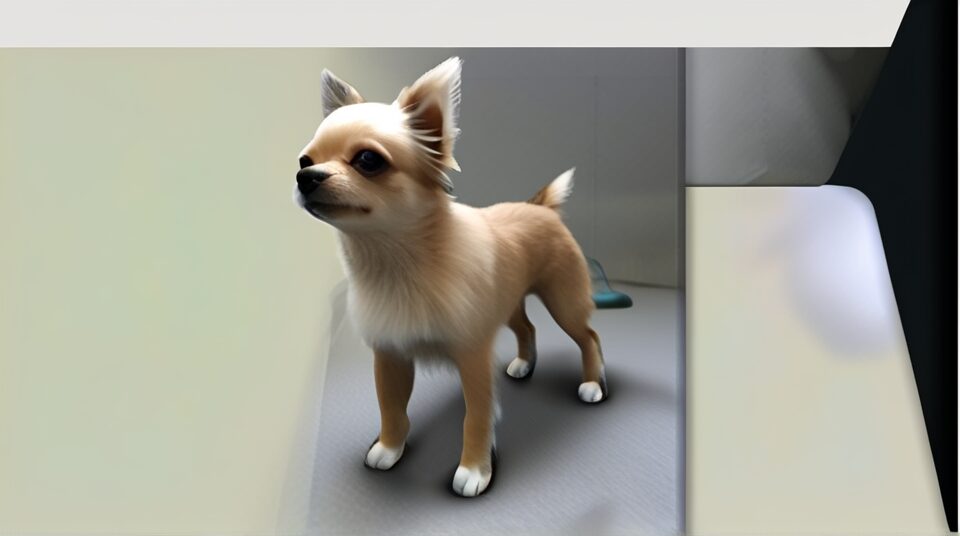
However once we started finding what felt like portrait similarity, in a controlled pose, the ball seemed like it had really gained momentum.
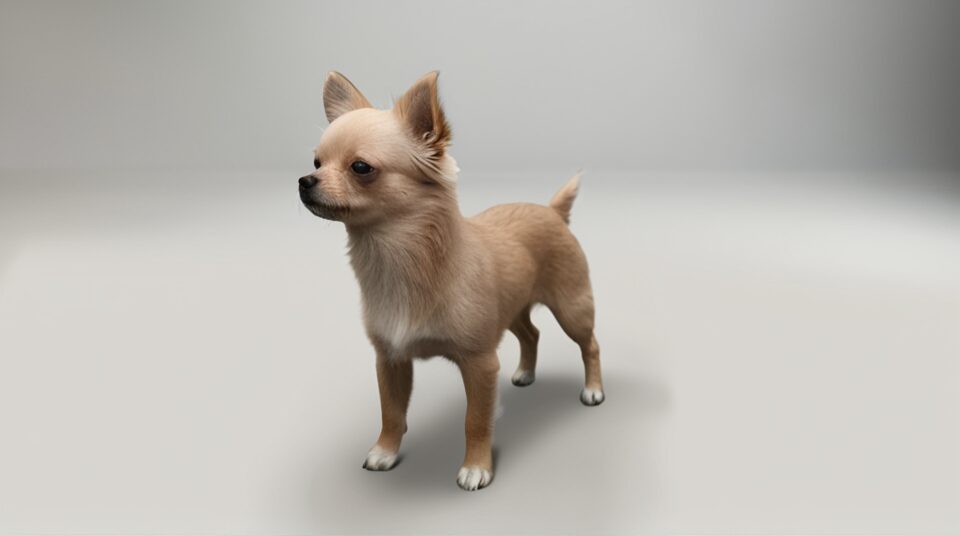
The improvements in the fidelity of our output would start to take huge steps, first from one month to the next, then one week to the next, and then from one day to the next.
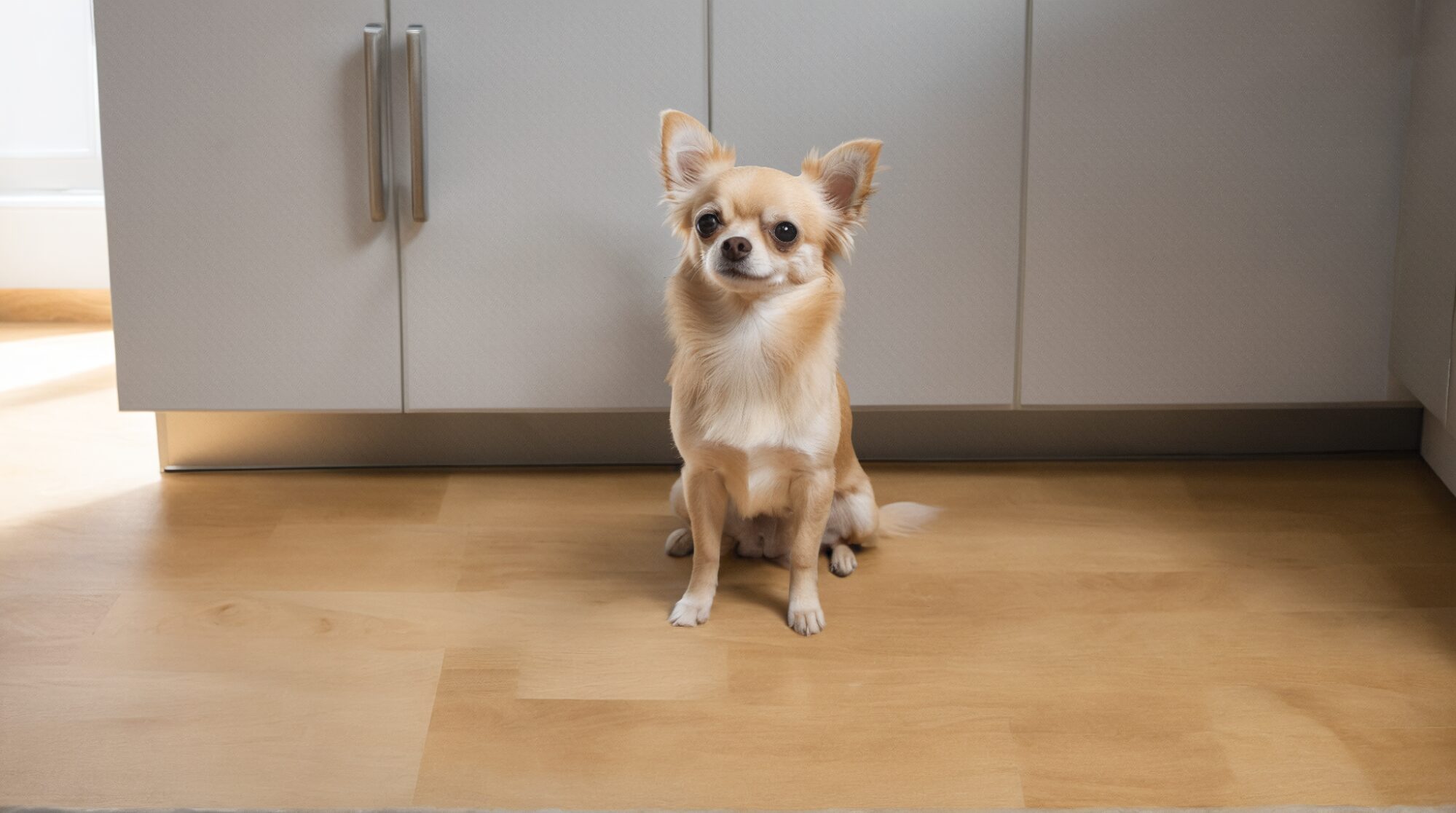
Skating to where the puck is going to be is the only way to engage properly with technology that moves as swiftly as AI. Once we had our plan and objectives clearly ready, we had to just go for it. There were days where new AI technologies would come out, and the best course of action for us would be to wait one day, to see if something else was about to overtake it, and to see if someone else had implemented it in a way that we could add it to our overall workflow easily. Other days we would find that some element of control we introduced had reduced the fidelity, and we needed a new way around it, adapting and iterating as we went.
Final Thoughts
This project was very special for a number of reasons. The technical challenges were interesting, big and somewhat unknown. However the biggest driver was definitely the ambition to do something good for the shelter dogs, and I can say everyone on the team put in a lot of extra effort to make this nearly impossible thing possible. I think it’s a great example of using AI for good and am very proud of the team and the results.
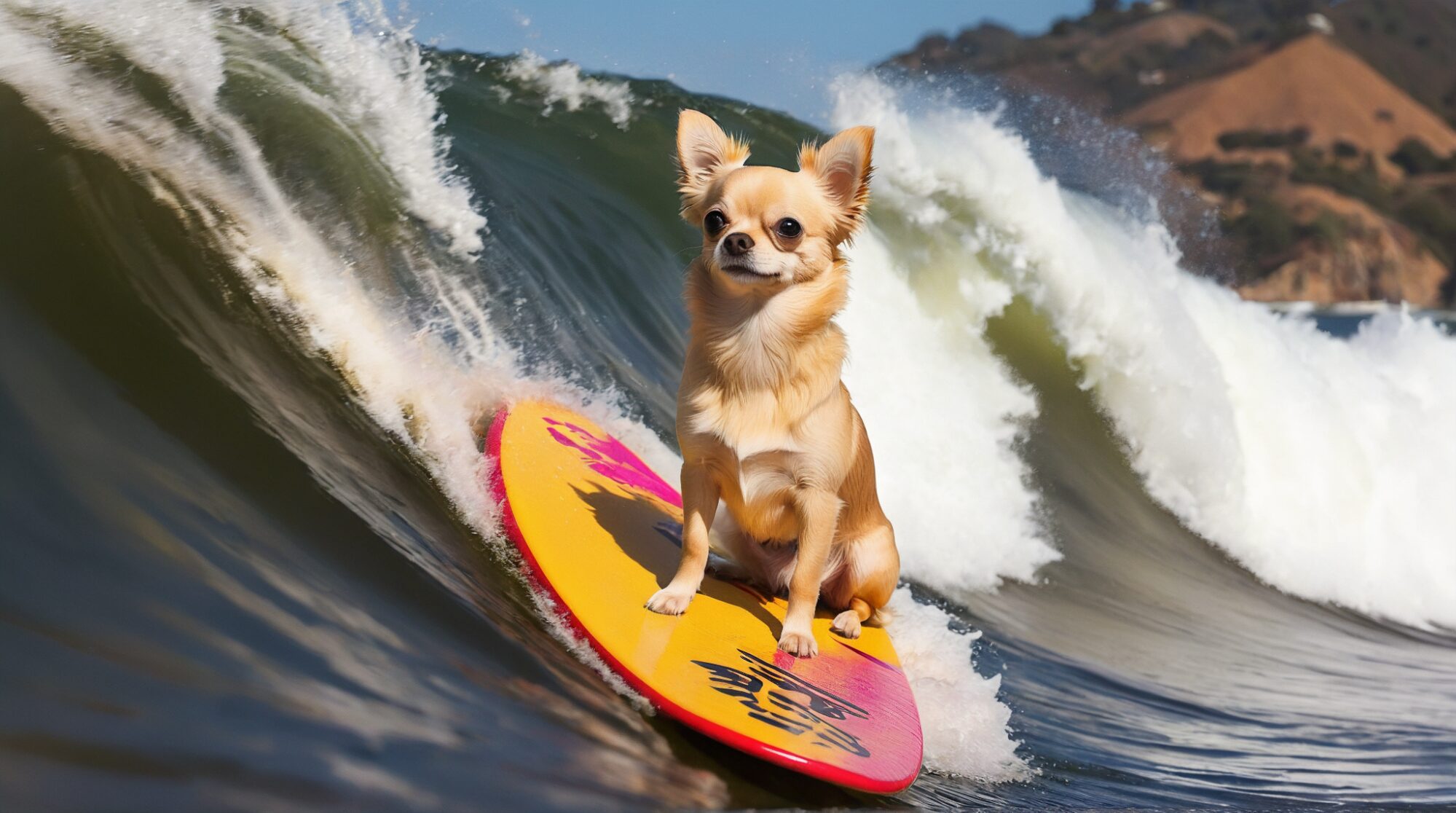
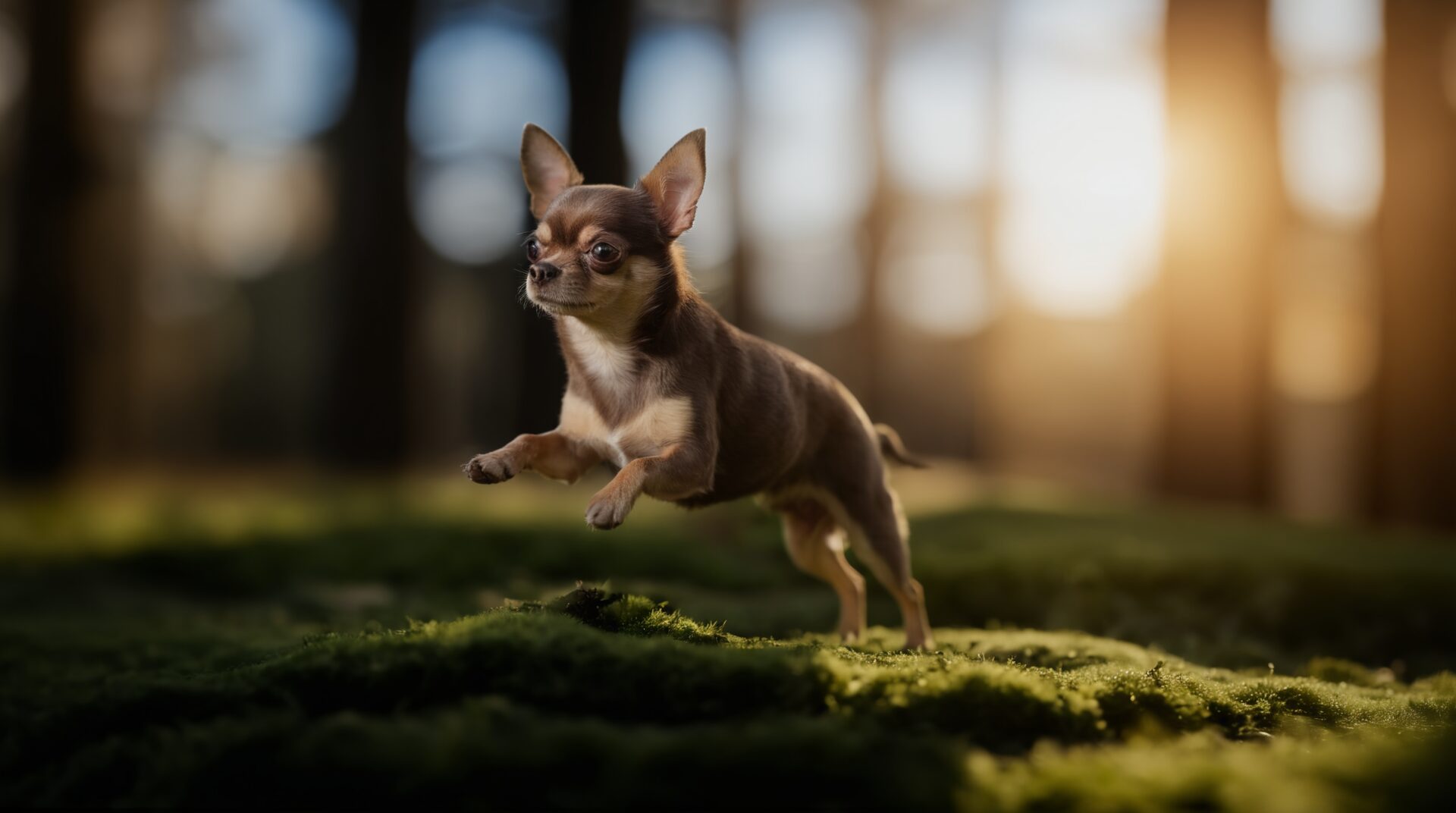
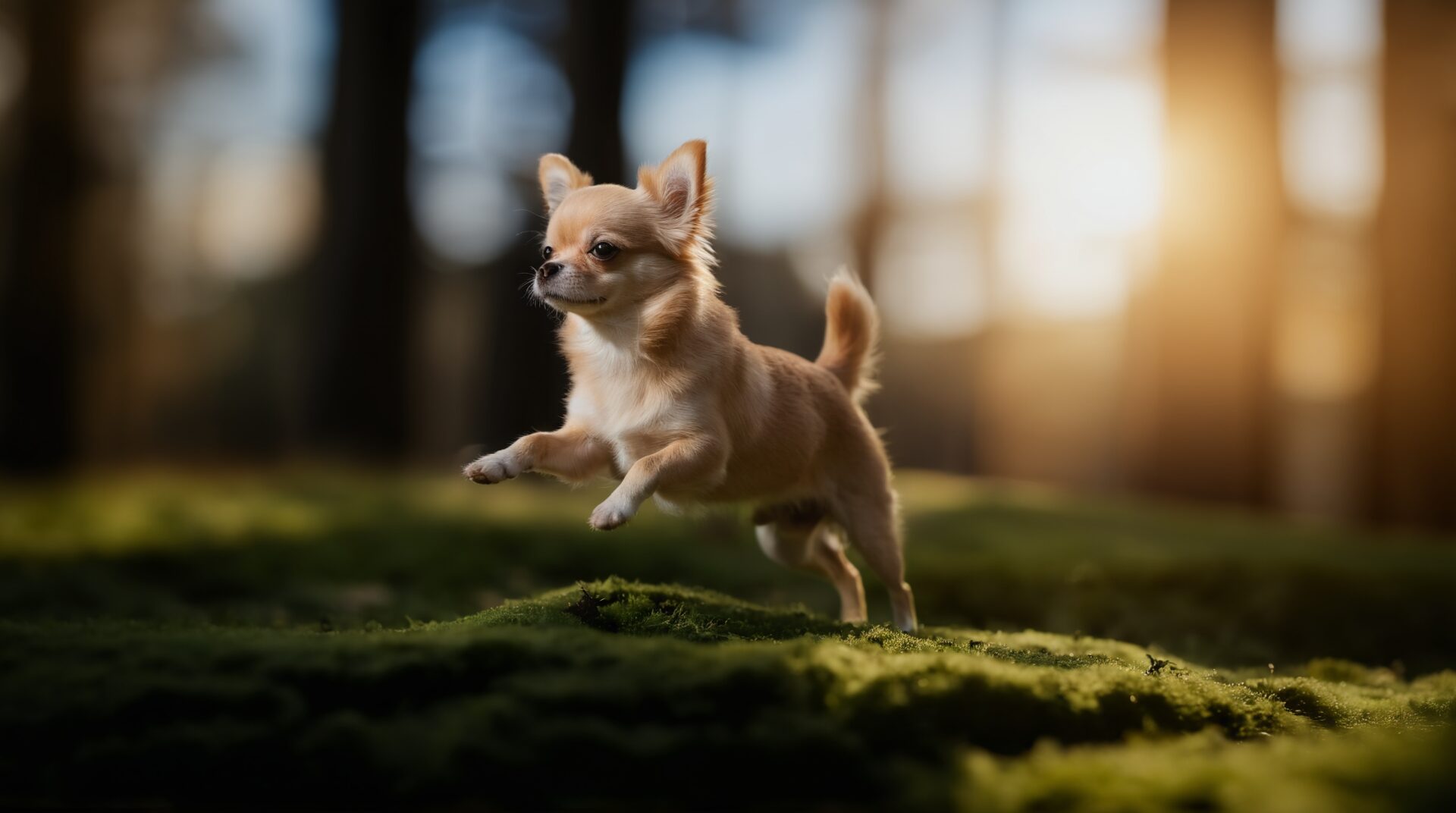
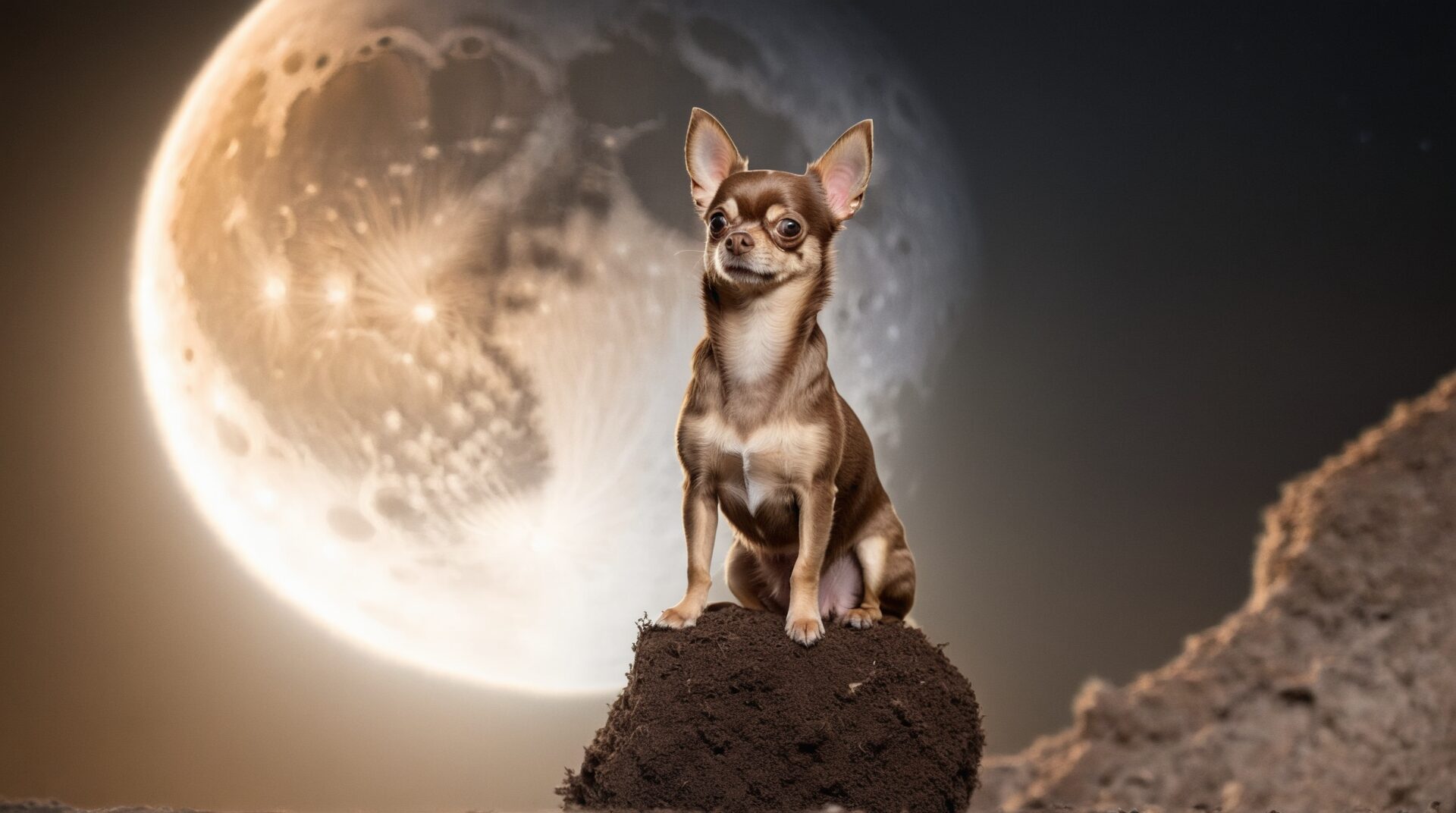